因此,在编程时对这两种误差有比较清醒的认识显得尤为必要。
Steven C. Chapra在”Numerical Methods for Engineers“一书中采用泰勒公式对此进行了分析,并给出了Matlab示例。
本人把这个例子转化为了下面的Fortran代码,供大家参考。
因为Fortran无法直接绘图,计算结果的图示是通过Gnuplot实现的。
该例子采用中心差分计算在x=0.5时函数f(x)=−0.1x^4−0.15x^3−0.5x^2-0.25x+1.2的一阶导数随步长的变化,其具体说明参见原书,在此不再赘述。
program www_fcode_cn implicit none integer :: i real(8) :: h,d,e,x,dftrue open(10,file='result') write(10,'(a)')'# step size finite difference true error' x=0.5_8 dftrue=dfunc(x) h=1 do i=1,11 d=(func(x+h)-func(x-h))/(2*h) e=abs(dftrue-d) write(10,'(f12.10,x,f17.14,x,f15.13)')h,d,e h=h/10 end do close(10) contains function func(x) real(8) :: x,func func=-0.1_8*x**4-0.15_8*x**3-0.5_8*x**2-0.25_8*x+1.2_8 end function func function dfunc(x) real(8) :: x,dfunc dfunc=-0.4_8*x**3-0.45_8*x**2-x-0.25_8 end function dfunc end program www_fcode_cn
计算结果文件result的内容为:
# step size finite difference true error
1.0000000000 -1.26250000000000 0.3500000000000
0.1000000000 -0.91600000000000 0.0035000000000
0.0100000000 -0.91253500000000 0.0000350000000
0.0010000000 -0.91250034999996 0.0000003500000
0.0001000000 -0.91250000349985 0.0000000034998
0.0000100000 -0.91250000003318 0.0000000000332
0.0000010000 -0.91250000000542 0.0000000000054
0.0000001000 -0.91249999945031 0.0000000005497
0.0000000100 -0.91250000333609 0.0000000033361
0.0000000010 -0.91250001998944 0.0000000199894
0.0000000001 -0.91250007550059 0.0000000755006
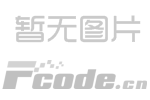
大图
从计算结果可以看出,当步长减小时,截断误差在减小,但当步长减小到一定程度后,舍入误差在增大。
因此,在实际计算时并非步长越小越好。在这个例子中,当步长在百万分之一左右时总体误差最小。